Research Overview & Publications
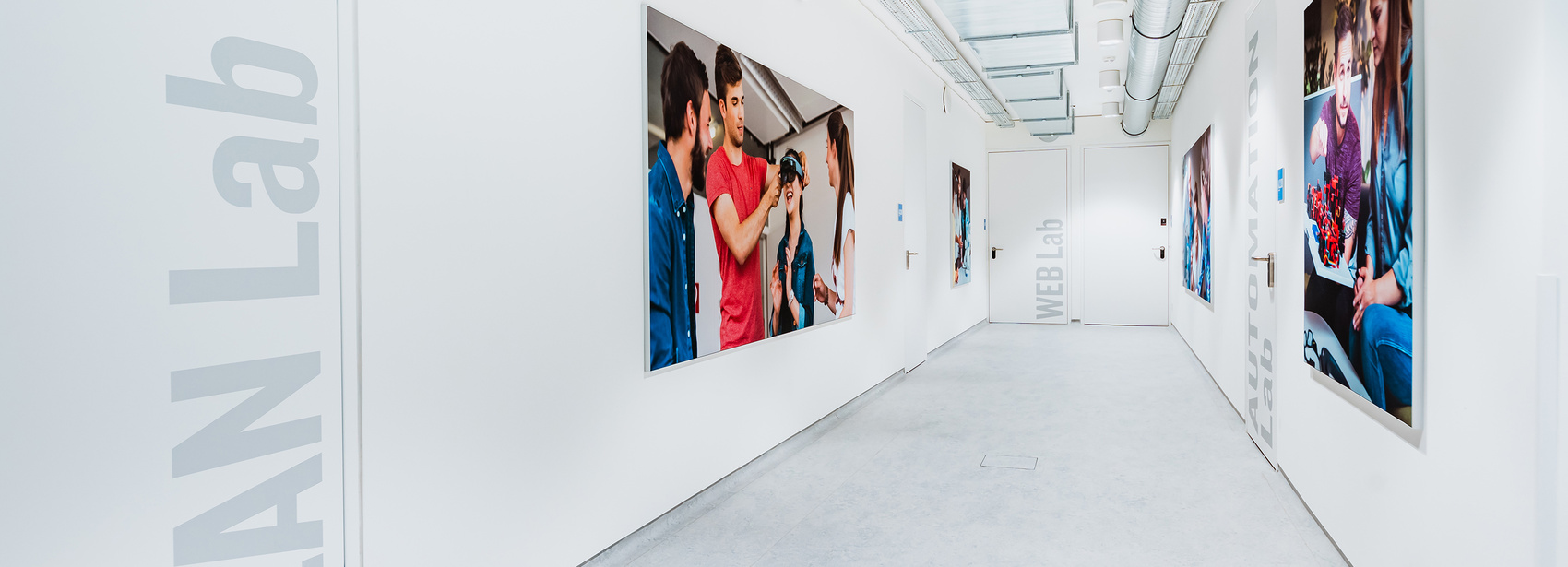
RESEARCH FOR DIGITAL AND SUSTAINABLE TRANSFORMATION
The FH Kufstein Tirol is an important regional educational and research institution that sees digital and sustainable change as central to social, cultural, economic and technological development. Our research focuses on the areas of technology, business and society.
Particularly in the areas of sustainability, digitalization, AI and international regional development, the effects of technological innovations on the economy and society are examined, whereby interdisciplinary approaches are required to overcome the challenges and opportunities of the 21st century.
Contact
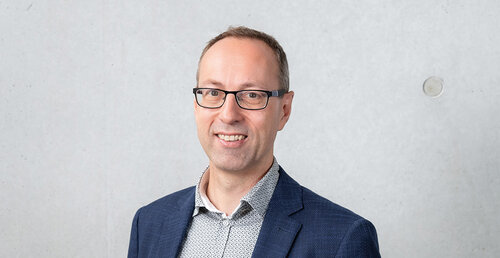
Prof. (FH) PD Dr. Mario Döller
Leiter F&E